Synthetic data sets – computer-generated samples with the same statistical characteristics as the genuine article – are growing more and more common in the data-hungry world of machine learning. These fakes can be used to train AIs in areas where real data is scarce or too sensitive to use, as in the case of medical records or personal financial data.
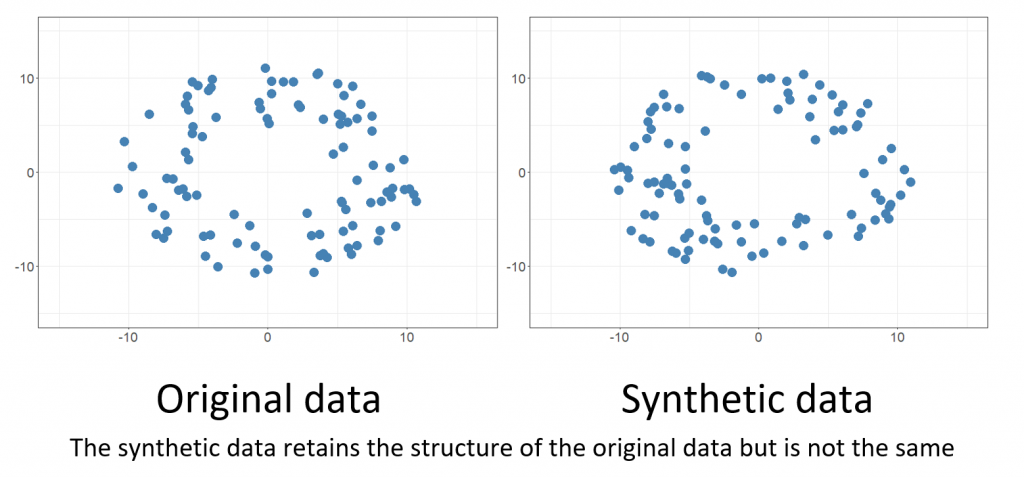
BY:
Salvatore Nicci
Technology Analyst / Reporter
PROJECT COUNSEL MEDIA
24 March 2022 (Rome, Italy) – I read “When It Comes To AI, Can We Ditch The Datasets?” The answer, as you may have surmised, is, “Absolutely.”
Synthetic data is like polystyrene. Great for building giant garbage islands and not so great for the environment. So trade offs. What’s the big deal?
The write up explains:
To circumvent some of the problems presented by datasets, MIT researchers developed a method for training a machine learning model that, rather than using a dataset, uses a special type of machine-learning model to generate extremely realistic synthetic data that can train another model for downstream vision tasks.
What can one do with made up data about real life? That’s an easy one to answer:
Once a generative model has been trained on real data, it can generate synthetic data that are so realistic they are nearly indistinguishable from the real thing.
What can go wrong? According to the article, nothing. Well, nothing might be too strong a word. The write up admits:
But he [a fake data wizard] cautions that there are some limitations to using generative models. In some cases, these models can reveal source data, which can pose privacy risks, and they could amplify biases in the datasets they are trained on if they aren’t properly audited.
Yeah, privacy and bias. The write up does not mention incorrect or off base guidance. But that’s close enough for nothing for an expert hooked up with IBM Watson (yes, that Watson) and MIT (yes, the esteemed institution which was in financial thrall to one alleged human trafficker).
And Dr. Timnit Gebru’s concerns? Not mentioned. And what about all the problems identified and detailed about synthetic data in Cathy O’Neill’s Weapons of Math Destruction? Not mentioned.
Hey, it was a short article. Synthetic data are a thing and they will help grade your child’s school work, determine who is a top performer, and invest your money so you can retire with no worries.
“Efficient”, “acceptable error rates”, “objective methods”. What could go wrong? No worries, right?
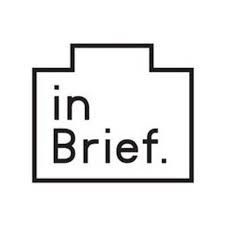
I do not have the space to make this one of our full-blown tutorials so I’ll just make a few basic points to give you the gist.
As noted in the most recent Gartner monograph, by 2024, 60% of the data used for the development of AI and analytics projects will be synthetically generated. In a separate study, nine out of ten technical decision-makers using vision datasaid synthetic data is a new and innovative technology and critical to staying ahead of the curve.
Each year, the world generates more data than the previous year. In 2021 alone, an estimated 74 zettabytes of data were “created, captured, copied, and consumed,” according to the International Data Corporation — enough to fill over a trillion 64-gigabyte hard drives.
But just because data are proliferating doesn’t mean everyone can actually use them. Companies and institutions, rightfully concerned with their users’ privacy, often restrict access to datasets — sometimes within their own teams. And when the Covid-19 pandemic shut down labs and offices, preventing people from visiting centralized data stores, sharing information safely was even more difficult. Without access to data, it’s hard to make tools that actually work.
Enter synthetic data: artificial information developers and engineers can use it as a stand-in for real data. Synthetic data is artificially generated by an AI algorithm that has been trained on a real data set. It has the same predictive power as the original data but replaces it rather than disguising or modifying it. The goal is to reproduce the statistical properties and patterns of an existing data set by modeling its probability distribution and sampling it out. The algorithm essentially creates new data that has all of the same characteristics of the original data — leading to the same answers. However, crucially, it’s virtually impossible to reconstruct the original data (think personally identifiable information) from either the algorithm or the syntheticdata it has created.
Synthetic data is a bit like diet soda. To be effective, it has to resemble the “real thing” in certain ways. Diet soda should look, taste, and fizz like regular soda. Similarly, a synthetic dataset must have the same mathematical and statistical properties as the real-world dataset it’s standing in for. It looks like it, and has formatting like it. If it’s run through a model, or used to build or test an application, it performs like that real-world data would.
So statistical similarity is crucial. But depending on what they represent, datasets also come with their own vital context and constraints, which must be preserved in synthetic data. Data lab researchers all use the example of a hotel ledger: a guest always checks out after he or she checks in. The dates in a synthetic hotel reservation dataset must follow this rule, too. They need to be in the right order.
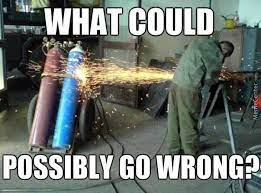
Demands for synthetic data are growing as the AI industry burgeons and there is a need for information to advance the technology. Efficient, acceptable error rates, objective methods: What could go wrong?
Well, while the benefits of synthetic data are compelling, realizing them can be difficult. Generating synthetic data is an extremely complex process, and to do it right, an organization needs to do more than just plug in an AI tool to analyze its data sets. The task requires people with specialized skills and truly advanced knowledge of AI. A company also needs very specific, sophisticated frameworks and metrics that enable it to validate that it created what it set out to create. This is where things become especially difficult.
Evaluating synthetic data is complicated by the many different potential use cases. Specific types of synthetic data are necessary for different tasks (such as prediction or statistical analysis), and those come with different performance metrics, requirements, and privacy constraints. Furthermore, different data modalities dictate their own unique requirements and challenges.
Proving the veracity of synthetic data is a critical point. The team working on the effort must be able to demonstrate that the artificial data it created truly represents the original data — but can’t be tied to or expose the original data set in any way. That’s really hard to do. If it doesn’t match precisely, the synthetic data set isn’t truly valid, which opens up a host of potential problems.
For example, let’s say you’ve created a synthetic data set to inform the development of a new product. If the synthetic set doesn’t truly represent the original customer data set, it might contain the wrong buying signals regarding what customers are interested in or are inclined to buy. As a result, you could end up spending a lot of money creating a product nobody wants.
A distant, but still real, ramification of improperly created synthetic data is the possibility of what’s known as member inference attacks. The whole concept of synthetic data is that it’s not in any way tied to the original data. But if it isn’t created exactly right, malicious actors might be able to find a vulnerability that enables them to trace some data point back to the original data set and infer who a particular person is. The actors can then use this knowledge to continually probe and question the synthetic set and eventually figure out the rest — exposing the entire original data set. Technically, this is extremely difficult to do. But with the right resources, it’s not impossible — and, if successful, the implications could be dire.
One potential problem with synthetic data that can result even if the data set was created correctly is bias, which can easily creep into AI models that have been trained on human-created data sets that contain inherent, historical biases. Synthetic data can be used to generate data sets that conform to a pre-agreed definition of fairness. Using this metric as a constraint to an optimizing model, the new data set will not only accurately reflect the original one but do so in a way that meets that specific definition of fairness. But if a company doesn’t make complex adjustments to AI models to account for bias and simply copies the pattern of the original, the synthetic data will have all the same biases — and, in some cases, could even amplify those biases.
Where is this all going? I’ll finish with a section from “The struggle (futility?) of controlling or regulating technology in the modern world”, a monograph written by our boss, Gregory Bufithis, which many of you have. If not, you can read the introduction for free by clicking here. He is commenting on Photoshop’s “Deep Fake” edition, plus some notes on GPT-3:
Photoshop just added a set of ML-based tools to allow you to manipulate images in new ways – to change the expression of a face in a photograph, for example. Technically impressive, but now everyone can fake any image or video even more than they could before. Obviously this is enormous in the context of legal investigations and journalistic investigations. Up to now, deepfake detection has relied on semantic and contextual understanding of the content, and the basic forensic methods available. But as I have noted in previous posts, it will get so much harder to detect these images. The more advanced technological developments in image manipulation currently underway via GAN models, plus synthesized video technology which combines video and audio synthesis together in one tool to create realistic voices, will make these images undetectable.
So the AI to create photorealistic faces, objects, landscapes, and video isn’t that far behind GPT-3 which can already write dialogue and articles (and movie plots) almost indistinguishable from ones written by humans. When OpenAI released its latest GPT-3 this past summer, it marked the first time a general-purpose AI saw the light of the day. But it also marked just the latest in a long history of knowledge technologies, the subject of my second monograph due next year.
And the level of abstraction? There is a running joke in Silicon Valley that says with GPT-3 and GAN models you can write a Taylor Swift song about Harry Potter. But soon, you’ll be able to make a new Harry Potter movie starring Taylor Swift.
Last year, the cyber firm Sensity revealed that a pornographic “deepfake ecosystem” has spread across the messaging app Telegram. Its researchers discovered that bots have created over 104,000 fake, AI-generated nude pictures of real women. Images were shared in private or public channels beyond Telegram, for “public shaming or extortion-based attacks.” The bot network was boosted on VK, Russia’s largest social media network. Telegram was unresponsive (read: had no reaction) when Sensity reached out with its findings.
How does Sensity do that? It blends “automated detection of visual threats” – via web crawlers and computer vision – with the work of threat intelligence experts, who search for malicious activity among underground communities on the dark web. Private groups and encrypted messaging apps pose a challenge to effective deepfake detection (and content moderation writ large). In the above case, Sensity uncovered activity in public channels by searching for keywords on Telegram. Since these deepfake services are not “illegal” or perhaps live in a grey area of the law, the bot network’s operators can freely promote and monetize their malicious service.
As I have noted in many posts: deepfake technology has been commoditized. Progress in synthetic media has been phenomenal in the last few years. 2020 was definitely the tipping point for these technologies to become pervasive, for the good but also for the bad. A few years ago, someone may have needed AI training to generate deepfakes. Now, they can leverage off-the-shelf synthetic data models for malicious or harmless purposes.
As I noted above, creating synthetic data is a very complex process, so organizations need to determine whether their data scientists and engineers are capable of learning how to do it. Creating synthetic data isn’t for the faint of heart. The sheer complexity associated with doing it right — and the potential pitfalls of doing it wrong — means organizations should be sure it will deliver sufficient value in return. But synthetic data is now at the cutting edge of data science, and more and more organizations are experimenting with how to get it out of the lab and apply it to real-world business challenges. We are seeing how this evolution will unfolds … and the timeline it will follow does not look good. I’ll end with Albert:
“All of our exalted technological progress, civilization for that matter, is comparable to an ax in the hand of a pathological criminal”
– Albert Einstein, 1917